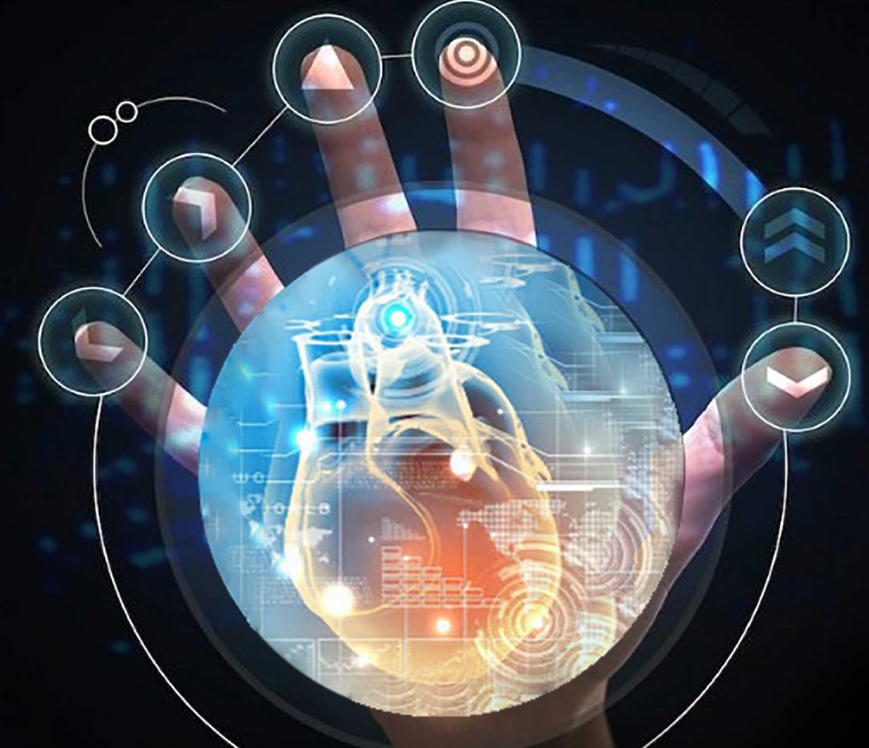
Our behaviors account for the most significant influence on overall health outcomes and healthcare costs, far outpacing genetic effects or access to medical care. And yet, many people have a difficult time improving their behaviors. As we move from an era of “reactive” medicine that is hospital and clinic-based toward more holistic and proactive care, we need new ways of helping people improve their behaviors and manage their health. This presentation will describe AI techniques for inferring individuals’ states in real-time from streaming sensor data and mobile interactions. Our health coaching infrastructure uses this framework for delivering tailored just-in-time motivational and feedbacks mobile messaging. This architecture incorporates computational models of user preferences, motivations, and barriers to change rooted in known principles of health behavior change. To reach our goal of helping users improve health behaviors, we use computational modeling, prediction, and optimization applicable to individuals in specific contexts and scenarios. This presentation will discuss examples of robust computational modeling and predicting individuals’ behaviors combining machine learning, hybrid dynamic systems, and statistical signal processing with psychological knowledge. We will illustrate how computational modeling can estimate cognitive functionality from unobtrusive observations of everyday behaviors combined with data from wearable sensors to optimize the interventions.
Images are licensed under Creative Commons License.